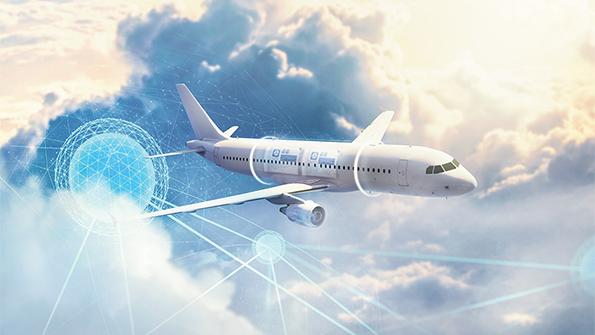
Big data, the Internet of Things, digital twinning and machine learning have become buzzwords in aviation and many other industries. But for aircraft MRO, the buzz must translate into new and beneficial forms of predictive maintenance to have practical value.
Of course, maintenance has already been at least partially predictive for a while. Engine health monitoring has long been a staple of engine support, cutting costs and keeping powerplants on wing longer. For nonengine components, solid reliability analyses can estimate probable failure rates after certain levels of hours or cycles have been reached, and planners can set inspection or removal rates based on these probabilities.
What is new is that the predictive process for nonengine components is moving closer to the engine model: that is, using multiple sensors and other data streams to make more accurate predictions for each aircraft part or system.
Rate of Adoption
However, these new techniques are still very much a work in progress. For example, Boeing is seeing continued growth in demand for its predictive tools, which include the work of data scientists as well as artificial intelligence and machine learning. But Tonya DelMaestro, chief marketing officer of Boeing Global Services, agrees with others that so far the serious users of these tools comprise only a small percentage of all airlines.
About 10% of Airbus’s A320 family is now on the OEM’s predictive maintenance program, according to the manufacturer.
Philippe Bordel, digital commercial manager of AFI KLM E&M, estimates that less than 5% of the world’s top 400 or so airlines have made any real progress with their predictive maintenance programs. Among airlines that have publicly stated that they are working on predictive maintenance, “most are co-developing their systems with a third party,” Bordel adds.
Frank Martens, head of customer development for digital products at Lufthansa Technik (LHT), says the number of airlines using predictive maintenance on nonengine components with the latest big data technologies is limited but growing fast. “We may be talking about 50 airlines worldwide,” he suggests. He distinguishes between mere prediction and predictive links to actual MRO actions, which he says LHT’s Aviatar platform provides.

Jean-Philippe Beaujard, general director of Revima’s Flightwatching unit, does not see most European airlines taking predictive maintenance to a high level. For his company, the carriers most active in using the new tools are in Asia and the Middle East.
“Asia is a very dynamic market, open to innovation in predictive maintenance and other forms of digitalization,” Beaujard says. Greater interest in predictive techniques in the Middle East stems from a harsh environment that presents more maintenance challenges. “OEM monitoring does not work well in the region, they get failures before other carriers,” he explains. Extreme heat and sandstorms are usually the culprits.
The number of carriers doing predictive maintenance is still “very limited,” says Yann Cambier, a senior consultant at ICF and a former AAR executive. He adds, “Most airlines are not sophisticated enough to implement the new processes required to exploit predictive maintenance technologies.”
Yet the rewards are real and benefits substantial. Cambier points to Delta Air Lines, which claims a dramatic reduction in maintenance-related cancellations using Airbus’s Skywise predictions for its A320s and A330s. Significantly, Delta’s error rate, or no faults found, was only 5%.
Airbus and Delta are now forming a digital alliance to develop new predictive solutions, with more data, to offer to other airlines. Airbus representative Martin Fendt says Airbus predictive maintenance reduces unscheduled events by 10-50%, depending on the components. The biggest improvements are for air conditioning and pressurization systems, electrical power, landing gear and brakes and pneumatics.
Boeing estimates that each removal of a component that is near failure saves several hundreds of thousands of dollars. Most of these savings are derived from avoiding revenue losses from schedule interruptions. Boeing Global Services Analytics Director Stefan Karisch says there are also savings from making a repair early, before a component fails.
The airframe OEM and its airline partners generally choose to work on components that are causing the most interruptions, rather than just the easy, “low-hanging fruit,” DelMaestro says.
Cathay Pacific Airways has adopted Honeywell’s Forge predictive solution for its A330 auxiliary power units. Honeywell estimates Forge can prevent about 35% of APU failures.
Forge also covers 10 other ATA chapters. For example, it can reduce delays and cancellations related to pneumatic components by 30%, with a no-fault-found rate of only 2%, says product director Josh Melin. For hydraulics, the results are even stronger, eliminating 80% of delay events and 40% of minimum equipment list events. False positives are less than 5%, Honeywell reports.
Digital manager Jacques Guichard estimates that AFI KLM E&M’s Prognos tool can prevent one flight cancellation per year for each ATA chapter of components it is applied to, not including delays avoided.
Martens says potential gains depend on the operational environment and MRO services used by airlines. For some components, reductions in unscheduled removals can reach 80%, while for some other components reductions are 30-40% and for others just 10%.
Beaujard says customers do not tell him how many unscheduled removals are being eliminated, but they are apparently very satisfied with the service. “Today in the Middle East, 100% of our recommendations are followed, so they have a high degree of confidence in them,” he observes.
Predictive results also vary with an aircraft’s age. For example, the Boeing 777 can generate four times as many alerts as the 737NG, and the 777X provides four times as many as the 777. But Karisch says Boeing is also working on predictions for older aircraft and notes: “There is a lot of operating data you can leverage from a 737. Adding sensors is not always the answer in the first place.”
Fendt explains that best-practice predictive maintenance processes are similar for previous and next-generation aircraft, because value comes from monitoring the life cycle of components, not of the aircraft itself. Airbus can retrofit older jets with FOMAX, an onboard data-capture and transmission module that boosts the parameters that can be captured on an A320 from 400 to 24,000.
“On the latest-generation aircraft, we can increase the number of sensors being monitored, which means more data is being fed back to our systems, thereby enabling us to define more efficient predictive maintenance algorithms,” Guichard observes. But Martens argues that it also makes sense to retrofit sensors on older aircraft. He stresses that nonsensor data is important as well, for example repair data from MRO shops.
“Older aircraft can be very interesting,” Beaujard says. For older Airbus A300s and Boeing 747s, Flightwatching must usually go onboard to get data. But with a flight-data acquisition unit installed, the company can use ACARS to query and receive data remotely. And sensors retrofit on bleed systems of older 737s can also improve predictions for these components.
But with all these benefits, why has progress toward predictive maintenance been so slow? Cambier asserts that the biggest hurdle to predictive maintenance is lack of engineering expertise: “Only a few players in the industry have the expertise to effectively implement predictive maintenance, decades of MRO expertise with large owned fleets or third-party contracts.” Other elements that must mature are data sharing, better and cheaper connectivity and a willingness to remove serviceable units.
Data Control
Martens agrees that data control is essential: “More and more OEMs restrict access to operational data for airlines, MROs and other players . . . this is not acceptable.”
Karisch says Boeing predictive maintenance always starts with a partnership discussion with the airline, which owns the operating data. “We decide what we want to accomplish, then work back to analytic issues and how much data is required,” he says. “It’s a partnership.”
Martens says lack of data interfaces within an airline also can hamper predictions, a problem Aviatar was designed to address. Pilot unions and work councils may restrict the use of data, while some governments also hamper data sharing.
Guichard acknowledges predictive maintenance drastically changes the way airlines work with OEMs and MROs. “Aviation regulations will also need to evolve, with regulators having to find a way to adapt to this new way of maintaining aircraft.”
Beaujard says another hurdle is that airlines often want quick results, but “it takes three to four months to solve a new problem.”
Airlines must be willing to share operations data with a provider because they trust that the data will be used to benefit the airline, says DelMaestro. Then airlines must trust predictions enough to change maintenance practices or proactively schedule component maintenance events in advance. Securing that trust requires holding no-fault-found, or false positive, rates to a minimum.
DelMaestro agrees that connectivity also can be a problem. Moving data off of aircraft can be tough if a carrier has a tight schedule. “Data is not always their highest priority,” she notes.
Fendt says airlines need to understand the technology, invest in it and modify processes and tools. “Data scientists should be integrated into the standard airline environment and data competencies added to the maintenance operations. Further, the value chain needs to transform its test-bench and no-fault-found policies to the predictive approach, to avoid in-service degradation of components.”
Many elements have to fall into place to make predictive maintenance work. But as experience with the process is gained, obstacles become easier to overcome. Despite data-sharing conflicts and other issues, progress is being made.