Next Steps For AFI KLM E&M Drones: Outdoor Inspections, Measuring Dent Depth
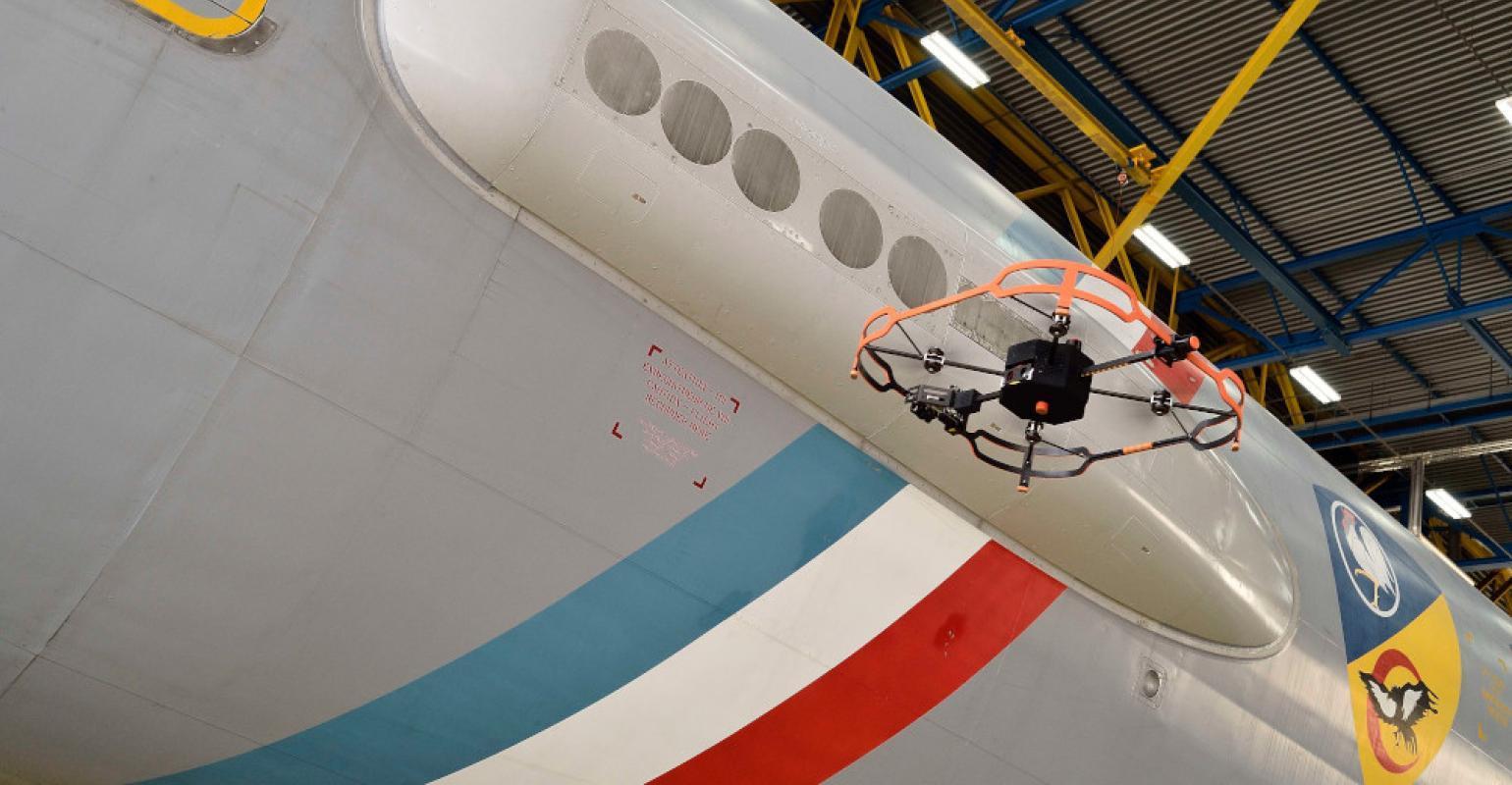
In 2016, AFI KLM E&M embarked on an important innovation, using drones for line MRO inspections.
The global MRO has completed four use cases for drones and is now using the devices to save time and improve efficiency on Airbus A320 inspections in Paris hangars, according to innovation director James Kornberg.
The next steps will be deploying drones outside hangars and using them to measure the depths of dents, predicts Josselin Bequet, CEO of AFI KLM E&M’s innovation partner Donecle.
Bequet and Kornberg reviewed their drone journey for participants in Aircraft Commerce’s recent Virtual Conference. The journey started in 2016, with the goal of saving time and digitizing inspections to increase traceability and improve efficiency.
Proofs of concepts were conducted in 2017 to determine what kinds of inspections Donecle drones could do. Next, drones had to be integrated with hangar planning and other operational constraints, and job cards generated for the several tasks drones would perform.
Kornberg chose several use cases to test, develop and deploy the drones. First was checking for placards and markings and streamlining ordering of new placards. Second was automated mapping of existing damage markings on aircraft and quickly comparing these with mapping data on the MRO’s ERP system.
Third came assessments of paint quality on fuselages to help prioritize the repaint program. And fourth was inspections for lightning damage, with concentration on potential damage areas.
The same basic Donecle technology is being used by other MROs, including AAR, LATAM and Austrian Technik. These drones can inspect an A320-family aircraft in 30-40 minutes with no pilot or GPS, Bequet explained.
Bequet said an iterative process, going step by step, is essential to successful implementation. His team had to learn how a hangar works and needed constant feedback from users to improve the drone’s image analysis and classification of damage types. Training and frequent assistance during implementation were also required.