Opinion: How To Demonstrate AI System’s Safety
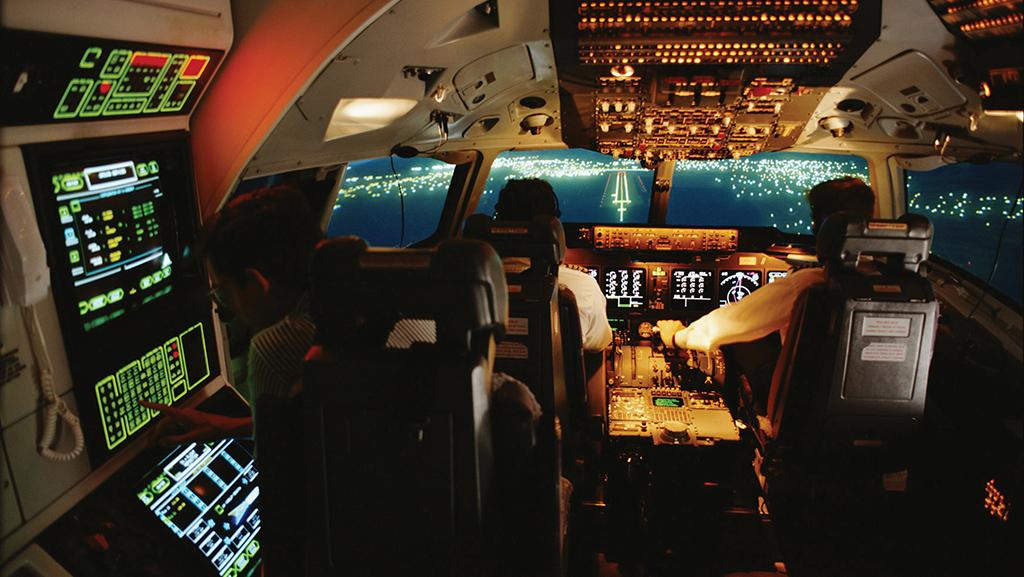
Artificial intelligence (AI) is heralded as the next great technological revolution to transform aviation. Already it is changing how we service aircraft by powering predictive maintenance solutions, and one day AI will transform flying itself. For instance, AI is seen as critical to enabling new types of aircraft such as drones and autonomous air taxis, and it is likewise essential to managing a fast-evolving and increasingly complex airspace.
However, AI is far from guiding aircraft today, and no one is about to step on board an autonomously piloted airliner. While automation has indeed been a function of airplane systems for decades, AI—which, unlike automation, allows for machine decision-making at a high level—has not yet crossed from the realm of the IT data center into the aircraft or air traffic control system (ATC). That is about to change.
The technology to fly an airplane autonomously with AI (considered a flagship use case for the technology) or to manage the airspace is robust. The issue is not technological maturation but one of certification. In our regulated environment, how do we demonstrate that an AI system performing a safety-critical task is safe and can be trusted?
In June of last year, leaders from the aerospace engineering community gathered to answer this critical question with the creation of a new standards effort focused on AI certification. Born out of this work is a new joint international committee, SAE G-34/Eurocae WG-114. This committee—which now comprises more than 500 engineers, scientists and research fellows from across the aviation ecosystem—is working to create a strong and well-supported means of compliance for AI certification by the autumn of 2022. This is in line with the European Union Aviation Safety Agency’s AI road map, released in January of this year, which calls for the first AI component to be certified by 2025.
The committee’s research of existing aerospace engineering standards concludes that there is no clear pathway to certify AI through an existing means of compliance when using the critical AI subdomain known as machine learning with neural networks. (Machine learning with neural networks is widely viewed as the best way to build advanced AI-powered systems, up to and including autonomous flight systems.) The central issue is related to the concept of AI “explainability,” which refers to the fact that, unlike traditional software code that can be read and logically understood, the inner workings of a neural network are an undecipherable mystery.
While R&D efforts are underway to make neural networks explainable, and while others are trying to find a new explainable approach to AI that proves just as powerful as neural networks, a consensus is forming that perhaps a better option is to devise a new approach to certification that works with neural networks as they are. Fortunately, we already have a useful model to work with: the certification of the human pilot.
When we bring a human pilot on a check ride, we do not wire diodes to the pilot’s head in an attempt to read the neurons firing in their brain. We would not even know what to make of that information. Yet if we certified pilots like we certify avionics systems, studying the neurons is what we would be required to do. Since we do not have any physiochemical way to judge that a pilot can safely fly an airplane, we devise tools like the check ride, flight school or ground-school curriculum, which we then leverage to assign trust that the pilot can safely fly an airplane.
This analogy makes for a useful road map for a supposed certification of AI. Determining what constitutes a qualified ground school is similar to a critical question the committee is working on: How do we determine that the data gathered to train an AI system is suitably representative of the real world? Likewise, determining what constitutes a robust check ride is similar to another question: What are the requirements of the simulators and testing procedures used to verify AI performance?
While no one is going to rewrite the industry’s approach to certification overnight, by tackling these critical questions, SAE-G34/Eurocae-WG114 is building a foundation that will finally bring AI to aircraft and ATC systems. The committee’s first publication—a gap analysis of existing standards and statement of concerns for the development of AI certification—will be released this autumn.
Mark Roboff is the general manager for aerospace transformation at DXC Technology and chairman of the SAE-G34 AI in Aviation Committee.
Comments
Thank you for such an insightful opinion piece. Your analogy of the human checkride is quite clever and very helpful. I am one of many eagerly awaiting the committee’s gap analysis.
For those who have not seen the EASA AI roadmap mentioned by Mark in this piece, the concept of “trustworthiness of AI” as an ethical construct underlying certification is a key theme. The document assumes essentially no AI knowledge and builds up to some deeper points.
Section 5 of the roadmap is particularly valuable to me as a safety professional, since it highlights potential AI applications for enhancing safety risk management. Here’s a direct link to the roadmap:
https://www.easa.europa.eu/sites/default/files/dfu/EASA-AI-Roadmap-v1.0.pdf
Antonio I. Cortés
Florida