New Intell Challenge Is Separating Wheat From Chaff
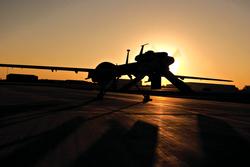
Credit: U.S. Army
With increasing numbers and types of sensors for airborne intelligence, surveillance and reconnaissance comes a dramatic increase in data collected. While attention is being paid to the means of collection, arguably the bigger challenge is how to cope with the mass of information once it has been...
Subscription Required
This content requires a subscription to one of the Aviation Week Intelligence Network (AWIN) bundles.
Schedule a demo today to find out how you can access this content and similar content related to your area of the global aviation industry.
Already an AWIN subscriber? Login
Did you know? Aviation Week has won top honors multiple times in the Jesse H. Neal National Business Journalism Awards, the business-to-business media equivalent of the Pulitzer Prizes.