Using Digital Twins And AI For Smarter Engineering

In today's engineering landscape, products across all domains have become increasingly complex and tightly integrated with sensors and automated control systems. This complexity presents both challenges and opportunities for organizations striving to optimize performance and efficiency. The primary challenge lies in ensuring that design, manufacturing, integration, and in-service support are interconnected, allowing each phase to learn from the others. This interconnectedness is essential for creating products that meet evolving demands and maintain high standards of quality.
On the other hand, the opportunity arises from the abundance of sensors and readily available computing resources. These sensors provide real-time data on a 24/7 basis for critical equipment, allowing for data to be shared, stored, accessed and processed efficiently. This influx of data can significantly enhance decision-making processes and operational strategies.
The Role of Digital Twins
Digital Twins are virtual replicas of physical systems that continuously evaluate operational impacts utilizing real-time data. They serve as dynamic models that facilitate a deeper understanding of system behaviors in operation and their impacts on performance and lifecycle. The key requirements for effective Digital Twins include:
Real-time Data Synchronization: This ensures continuous updates from physical assets to their digital counterparts, enabling immediate insights and proactive interventions based on current operational data. Maintaining accuracy and relevance is crucial for the digital model to reflect the actual conditions of its physical counterpart.
Data Management: Effective data management encompasses the collection, transmission, storage, and retrieval of data from physical assets. This capability is essential for providing real-time information about performance, conditions, and maintenance needs.
In engineering contexts, Digital Twins offer the possibilities of real-time diagnostics and prognostics, enabling impact assessments for off-design operations and facilitating maintenance scheduling for cause. Digital Twins being tightly integrated multi-disciplinary models, enable scenario modelling and evaluations. The off-design scenarios could, when data is available, be based off real time operational data. In defense applications, they enhance mission planning, asset management, and operational readiness by enabling simulation of various scenarios and outcomes. By leveraging these capabilities, organizations can optimize performance and extend the lifecycle of their assets while improving decision-making processes across various industries.
The Impact of Artificial Intelligence
Artificial Intelligence (AI) plays a pivotal role in engineering automation by enhancing data analysis and decision-making processes. AI excels at processing large datasets, identifying patterns, and providing actionable insights that significantly improve decision-making while automating routine engineering tasks for greater efficiency. By leveraging AI's capabilities, organizations can streamline operations and reduce human error.
One of the most impactful applications of AI in engineering is predictive maintenance. AI algorithms can predict equipment failures by analyzing real-time data from sensors, optimizing maintenance schedules, thereby reducing downtime and operational costs. This proactive approach not only enhances overall system reliability but also extends the lifespan of machinery by addressing potential issues before they escalate into costly breakdowns. Industries utilizing AI for predictive maintenance have reported significant reductions in maintenance costs and improved asset uptime.
Numerous defense engineering projects illustrate the successful application of AI for various purposes such as threat detection, logistics optimization, and mission planning. These implementations demonstrate enhanced accuracy and efficiency through intelligent automation, showcasing how AI can transform operational capabilities in high-stakes environments.
Synergy Between Digital Twins and AI
The synergy between Digital Twins and AI further amplifies these benefits. AI enhances the functionality of Digital Twins by enabling predictive analytics that allow for real-time simulations and scenario modeling. This combination improves operational insights and decision-making capabilities significantly. By integrating AI with Digital Twins, organizations can conduct advanced simulations that forecast potential issues and outcomes, facilitating proactive strategies that bolster overall system performance and resilience.
Moreover, this integration streamlines design processes and lifecycle management by providing continuous feedback based on real-world performance data. Successful projects have illustrated the effective use of Digital Twins and AI in optimizing manufacturing processes while enhancing system reliability through data-driven insights. This collaborative approach not only improves operational efficiency but also fosters innovation in engineering practices across various sectors.
Despite their potential benefits, implementing Digital Twins and AI presents several significant challenges that organizations must navigate effectively:
Data Security and Privacy Concerns: The integration of these technologies brings significant challenges around cybersecurity, privacy, and data integrity. With sensitive information at the core, protecting against unauthorized access and cyber threats is paramount. For organizations, cybersecurity demands a thorough assessment of potential threats and the establishment of effective deterrents. Additionally, data integrity must be ensured through clearly defined policies and roles related to data ownership and stewardship across various levels of the organization. When equipment is shared among different OEMs, data segregation for each becomes crucial to maintain security and privacy.
Technical Challenges: Organizations face numerous technical hurdles when integrating these advanced technologies. Compatibility issues between existing systems, complexities in data management, and the need for sophisticated infrastructure can impede successful implementation.
Need for Skilled Personnel: The successful deployment of Digital Twins and AI requires a workforce proficient in these areas. This highlights the importance of training programs to equip personnel with necessary skills.
We at Quest Global are involved in ongoing MBE (Model-Based Engineering) strategy development and deployment for an aerospace customer. This engagement is giving us valuable insights into the solution, technology and enterprise level considerations for digitalization. All solutions are customized through close collaboration with customers to align with their vision of a digitally integrated product delivery and support organization. Quest Global also has capabilities to encompassing the digital engineering space from MBSE, model based definition to digital manufacturing, digital factory, and sustainment. These competencies have been successfully deployed across the aerospace and defense sector. Examples include digital twins for aerospace manufacturing, model based design and definition for aero engines, covering conceptual design, design space exploration, optimization, and 3D analysis leading to model-based definition.
Future Trends
Looking ahead, the evolution of Digital Twins and AI in engineering is expected to bring about more sophisticated systems that enable deeper insights and greater automation. This transformation will significantly impact operational strategies across various industries—particularly in defense—where innovative applications could enhance situational awareness.
Industry stakeholders are urged to invest actively in these technologies. By fostering collaboration and innovation, organizations can harness the full potential of Digital Twins and AI to remain competitive in an ever-evolving technological landscape.
The integration of Digital Twins and AI offers transformative benefits across engineering sectors by enhancing efficiency, decision-making, and operational effectiveness through real-time data analytics. Embracing these technologies is crucial for organizations aiming to stay ahead in a rapidly changing landscape; it enables them to adapt effectively to new challenges while seizing emerging opportunities. As industries continue to evolve towards greater complexity, leveraging these advanced technologies will be key to achieving sustainable growth and innovation.
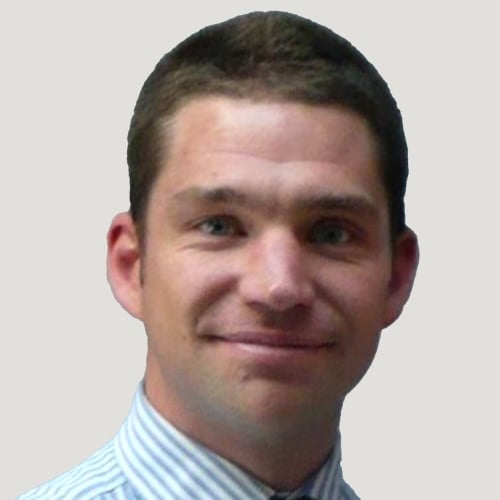
by Nick Charles - UK Defence, Quest Global
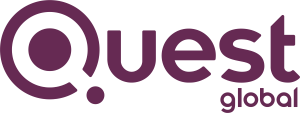